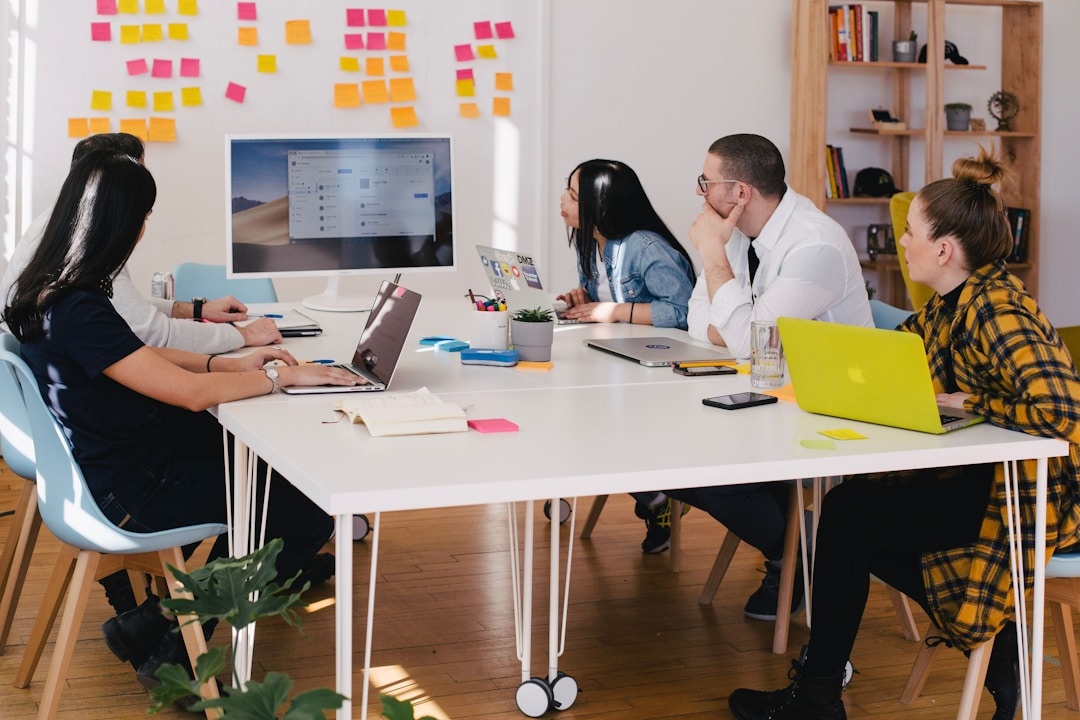
In the rapidly evolving digital age, organizations seek groundbreaking methods to streamline information flow and leverage data-driven decision-making. Artificial intelligence (AI) knowledge management systems have emerged as a vital tool in this transformative journey, enriching businesses with unparalleled efficiency and insights. However, their introduction and integration within an organization’s infrastructure pose unique challenges that necessitate strategic planning and robust solutions. This article delves into the complexities, barriers, and best practices of deploying these advanced systems. Keep reading to discover key insights for successful AI knowledge management implementation.
Understanding the Complexities of AI Knowledge Management Systems
Alt text: A team in an office discussing AI knowledge management during a meeting
Integrating AI into knowledge management presents a multifaceted challenge for many organizations. At its core, AI knowledge management aims to harness machine learning and natural language processing to organize, search, and deliver information effectively. This technology’s intricacies require those implementing it to have a firm understanding of both the AI’s capabilities and the nuanced needs of their business operations.
Another level of complexity arises from the need for these systems to interact seamlessly with existing IT infrastructures. Aligning AI algorithms with legacy systems and databases is an intricate task, often demanding extensive customization. To ensure long-term viability, stakeholders must consider elements like system interoperability and the future-proofing of their technology stack.
Security and privacy also dominate the list of complexities. AI systems must be designed to protect sensitive information while complying with data protection regulations. These regulatory considerations can significantly influence how AI platforms are structured and operated, further complicating their integration.
Overcoming the Barrier of High Costs in AI Knowledge Management
Cost is a significant barrier to the adoption of AI knowledge management systems. The expenses associated with deploying these systems can be prohibitive, starting from the initial investment in technology to the ongoing costs for maintenance and updates. Additionally, the specialized human capital required to operate and manage AI systems can add a hefty sum to the overall budget.
However, there are ways to mitigate these financial challenges. One approach is to prioritize modular and scalable solutions that allow businesses to start small and expand capabilities as needed. Choosing open-source AI technologies can also significantly reduce upfront software costs, freeing up resources for other critical areas.
Optimizing existing resources is another cost management strategy. Leveraging in-house talent and promoting cross-training can minimize the need for external hires. Organizations can also explore partnerships with AI service providers that offer pay-as-you-go models, which can be more manageable financially than large one-time expenditures.
Ensuring User Adoption and Ease of Use in AI Systems
Alt text: A woman in an office researching AI knowledge management on her computer
When implementing an AI system, user adoption is a critical success factor. Introducing new technology can often be met with resistance from staff who are accustomed to existing workflows and tools. Hence, it is vital to prioritize user-friendliness and intuitive design to encourage broad adoption across an organization.
Training and support play a significant role in easing the transition to an AI-driven system. Tailored training sessions that address particular user needs and concerns can improve confidence and competence with the new technology. Providing continuous support is equally important to promptly address any issues that users may encounter.
Integration into daily workflows is another focal point. AI systems should be seamlessly woven into the organization’s standard operating procedures. This means ensuring the system complements rather than disrupts existing processes, leading to genuine productivity and improvement in job satisfaction.
Feedback mechanisms are also essential for refining the user experience. Soliciting input from all levels of staff can provide valuable insights into how the system functions in real-world scenarios. This feedback allows for ongoing improvements, making the AI system an ever more effective and user-centric tool.
Overall, the successful implementation and maintenance of AI knowledge management systems demand a multifaceted approach. Thoughtful strategies must be used to navigate challenges such as data quality, cost, ease of use, and ongoing system maintenance. With the right framework and attention to detail, businesses can harness AI’s transformative power, enhancing their knowledge management and gaining a competitive edge in the digital landscape.